OPPORTUNITIES
& RISKS
Navigating the rapidly changing landscape of AI
Any discussion about AI must consider the risks presented by the technology. Proven and potential benefits of AI are also an increasingly central part of national and international conversations about major policy and societal challenges and how we might address them. The ways in which key opportunities and risks were presented in the roundtables were illuminating and suggested certain shifts and reframings that could strengthen governance and collaboration.
Now is the time to develop policy frameworks and ideas that work
The sense that there’s a window of opportunity to influence policymaking emerged strongly from almost every roundtable. To an unusual degree, government officials at the highest level are deeply engaged in questions of AI governance. The call to action to civil society, academia and those creating the technology
was clear.
“AI needs to help reframe the paradigm,
not support it.”
Royal Society of Arts, AI and the Future of Learning Roundtable Report
At the same time, balancing appropriate urgency with interrogation will be key to creating strong and inclusive policies. Particularly in the case of the sector and topic-specific roundtables, participants emphasised that rushing to apply AI to complex systems without fully understanding the historic challenges and dynamics that characterise these systems is a recipe for disaster. Education, food security and disability in the workforce are complicated topics, with challenges and intricacies that predate the advent of AI-powered technologies. Likewise, the forces that make the global data landscape unequal, or contribute to a possible stalling in ‘disruptive’ scientific research, are multifaceted and can’t be entirely addressed by AI. Additionally, while many of the challenges and opportunities of AI are new, sectors like healthcare and education have grappled with the integration of technological advances before. It’s important to engage with and learn from the past, as well as recognising the ways in which AI is novel.
AI is most often an enabler, not the solution itself
For most individuals and groups, AI is one strand in a web of social systems that impact their lives and opportunities. Applying AI to systems that are fundamentally broken won’t lead to equitable outcomes. As one roundtable participant put it, “AI needs to help reframe the paradigm, not support it.”
In several contexts, participants advised that we focus on the potential of AI to enable solutions and fuel progress, rather than viewing it as the answer to long-standing and complex policy challenges. Another roundtable participant emphasised that, “AI is only a tool, not a magic bullet.”² Likewise, AI was often framed as a complement to human intelligence and capabilities, as opposed to a replacement. One roundtable participant suggested that, in the context of scientific discovery, “greater interoperability between human intelligence and machine intelligence would unlock the potential to scale AI research projects and embed them within the research process.”
“We need to shift from thinking, ‘AI is the future,’ to ‘AI will help us get to
the future we want.’”
British Science Association, Untapping the Potential of AI in Science Roundtable Report
In education, AI-powered tools can help teachers by freeing them from administrative burdens and allowing them to focus on the aspects of education that they alone can provide to students.
By improving accessibility and removing current barriers to participation, AI could enable a world where everyone can bring their unique skills and perspectives to the workplace, regardless of disabilities.
In science, AI can be a transformative tool for scientists, especially when thought of as a “co-pilot.” While the potential impact of AI on scientific research is more fundamental than a better microscope, for example, it is still most productive to think of AI as a complement to human scientists, and to focus on maximising the complementarity of the relationship.
In climate, AI could play a pivotal role in forecasting early warning systems and monitoring complex dynamics, from natural disasters to food security challenges. Working alongside governments and civil society organisations, these insights could lead to the development of better response policies and processes for prevention and mitigation of environmental, market and systemic challenges.
AI developers, policymakers and civil society organisations alike could further orient their ‘north stars’ to the societies they want to help build and contribute to, as well as the technologies they can discover and the risks they need to manage. This paradigm shift would help optimise for beneficial outcomes for all.
2 Gro Intelligence., ‘AI and the Future of Food Security’
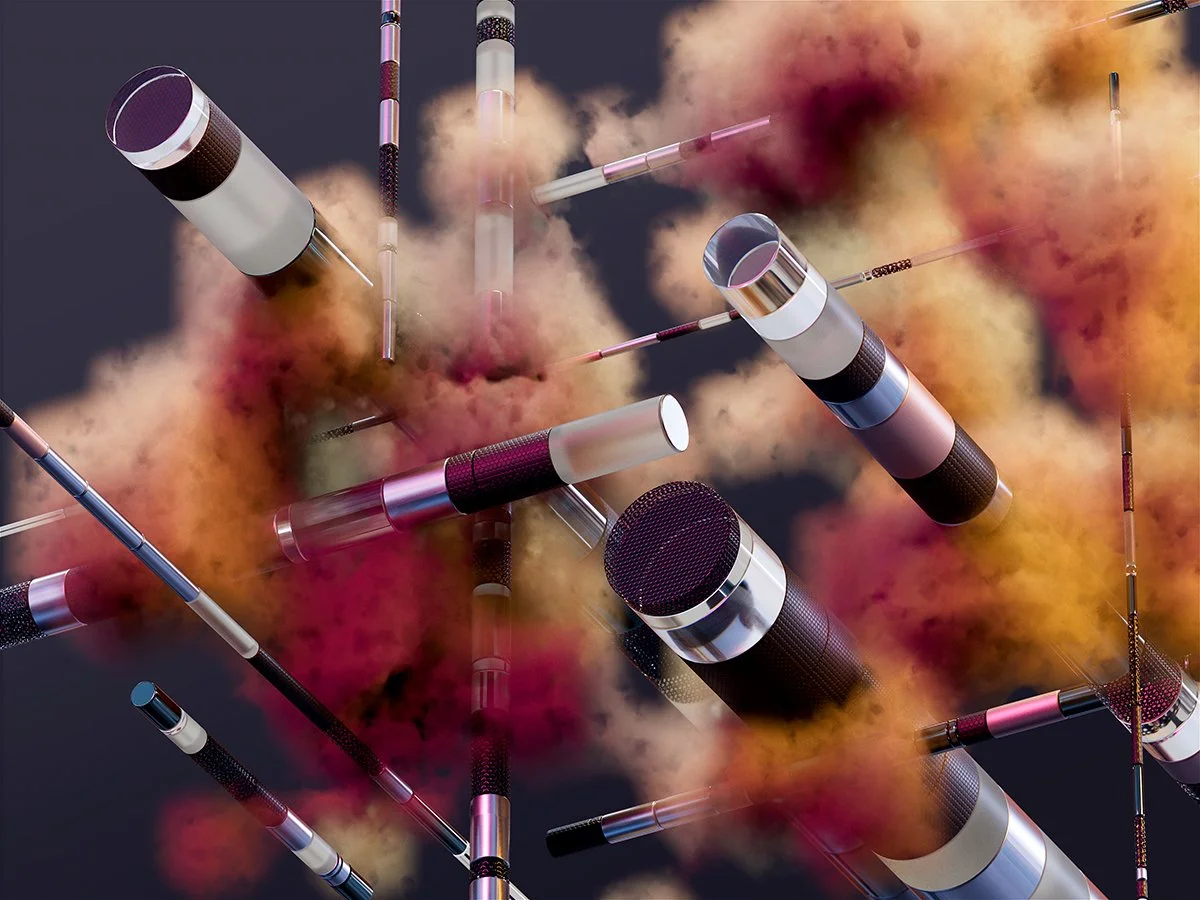
AI systems risk replicating and exacerbating existing biases, power imbalances and systemic inequalities…
Examples of biassed machine-learning outputs and the harm they cause, particularly to historically marginalised groups, are well established. Broad societal risks, including bias, misinformation, surveillance and inequitable access to benefits, were prominent in all the conversations. For example:
In workplace contexts, well-intentioned deployment of AI systems and tools could exacerbate existing barriers for people with disabilities if not rigorously tested with the involvement of those they are intended to help.
In scientific research, disparities in access to data and training will lead to unequal benefits from scientific progress - among scientists and societies alike.
Education practitioners highlighted the risks of applying AI too hastily to student assessment, as automated systems may reinforce biases inherent in the training data and impact students’ university prospects.
Throughout the discussions, participants pointed to the continued existence of a global digital divide. At the food security roundtable, it was emphasised that the economics of AI do not always promote equity of access. One participant asked how we might create the right market to scale solutions
to lower costs for participating in the use of AI.
Risks that AI will exacerbate existing inequalities are closely linked to questions of data equity. The datasets currently available for training AI systems are not fully representative of the global population and historical biases are often baked into the data used to train models. Data is neither generated nor collected at the same rate, or to the same standard, globally. Access to data, as well as its quality, can create and reinforce power imbalances. Not all data is publicly available and even when it is, availability does not guarantee accessibility. From nonstandard data schemas to lack of data legibility and literacy, there are lots of reasons why data may not be usable. Many existing initiatives designed to diversify datasets are currently operating only on small scales, while other collaborative data governance efforts only go some way to solving the issue.
Participants noted that loss of privacy is a significant near-term risk from higher participation in AI technologies. The risk that data might be misused in a way that betrays individual characteristics to that person’s detriment, or to a company’s outsized gain, surfaced throughout our discussions.
“While some risks will be evident from the capabilities
of the models themselves, many more will result from
the way those models interact with their environments and society at large.”
Centre for Security and Emerging Technology (CSET), Skating to Where the Puck is Going: Anticipating and Managing Risks from Frontier AI Systems Roundtable Report
…But AI can also address inequalities, if designed with that goal in mind
As noted, the fact that AI-powered systems and technologies entrench existing biases and inequalities featured prominently in the roundtable discussions.
Conversely, it emerged that there are ways in which AI could help increase equity – but only if developers, policymakers and citizens make that a goal. With good intentions, all groups risk misrepresenting the hopes and concerns of affected communities if they fail to engage directly with those communities. A propensity to make assumptions, and for those assumptions to gain traction in the debate, was evident. Sometimes the policies that would actually promote equity are counterintuitive. Conscious and active efforts must be made to realise the potential for AI to support, rather than undermine, equity.
For example:
AI could increase employment and earnings for people with disabilities, making the workforce more inclusive. By developing tools that increase targeted recruiting of people with disabilities or that help disabled job-seekers find the right opportunities, AI powered tools could also improve a disabled employee’s experience on the job.
Personalised learning could help level the playing field for children and adults alike, improving educational outcomes across the board. Generative AI opens up the potential for more genuinely personalised learning than we’ve seen in the past, while large AI models offer scope to improve the capabilities of AI-powered tools for teachers.
Re-thinking the collection, governance, architecture and management of data could unlock benefits across virtually all applications of AI. Within science, for example, data is the key difference between fields that use AI and those that don’t. To date, structural biology and genomics have led the way in terms of AI-enabled advances, partly because the life sciences have more established experience and frameworks for dealing with data. Many other domains, from materials science, physics and chemistry, to healthcare and criminology, have lots of unstructured data and making that data accessible and usable could hold the key to AI-driven advances in those fields and more.
AI could help democratise access to expert knowledge, ensuring that no one is left behind due to the inability to afford expert support. A participant in the food security roundtable highlighted the example of small scale farmers in India applying for subsidies. To apply, farmers need to read and complete long legal documents, which they often don’t have the literacy and/or legal knowledge to understand and are therefore excluded. AI could help address this challenge by supporting farmers to understand and complete their applications.
DEFINING DATA EQUITY
There are many definitions of data equity. One way to conceptualise it is as “a set of principles and practices to guide anyone who works with data (especially data related to people) through every step of a data project through a lens of justice, equity, and inclusivity. And equity is not just an end goal, but also a framing for all data work from start to finish.”
Safety risks associated with emergent capabilities are inherently challenging to manage
At the CSET roundtable, we proposed fi ve ways in which existing AI systems are currently being augmented that give rise to concern - namely multimodality, tool use, deeper reasoning and planning, larger and more capable memory and increased interaction between these systems and users.⁴
Of these, tool use was considered by some to be the most concerning near term capability, in part because of “the wide array of potential actions it enables, as well as the potentially high stakes and unpredictable outcomes of those actions.”⁶
AI systems could also be used to enable adversarial attacks. The potential for AI to gain advanced cognition skills (e.g. long-term planning or error correction) and to develop situational awareness (e.g. an awareness of its own testing, development or deployment) were both explored. Biosecurity risks could result from an AI system being given knowledge about biological production, and in the most extreme scenario, an AI system could develop novel synthetic weapons.
“By necessity, the concerns being raised are speculative, since they relate to the development of novel capabilities that have only been observed in primitive forms. However, waiting to take action until it is definitively proved that AI systems do have the capabilities under contemplation would be irresponsible, given the potential severity of the harm that could result.”
CSET, Skating to Where the Puck is Going: Anticipating and Managing Risks from Frontier AI Systems Roundtable Report
4 These five points are drawn from material presented by Matthew Botvinick (Google DeepMind) at the Centre for Security and Emerging Technology roundtable.
6 Centre for Security and Emerging Technology., ‘Skating to Where the Puck is Going: Anticipating and Managing Risks from Frontier AI Systems’.
Each of these capabilities has advantages, including the potential to make AI systems more useful, transparent or benefi cial. But downsides and risks were identified in relation to each. It is important to be vigilant in monitoring these augmentations, including in the context of the environments in which they operate. As the CSET roundtable report points out, “model capabilities that may seem concerning may in fact be harmless — for instance, if a model produces instructions on how to create a chemical weapon, but the necessary reagents are strictly controlled. On the other hand, ways in which a model may seem too limited to cause harm may be misleading — for instance, if a model’s context window is too short to develop and carry out a mass spear-phishing attack in one go, but tool use and memory allow the model to call external programming libraries, save fi les to refer back to later.”⁷
DEFINITION: MULTIMODALITY
A multimodal AI system is one that is capable of receiving multiple types of input (such as text, images, audio, or video) or generating multiple types of outputs.
DEFINITION: TOOL USE
Tool use refers to the capability of AI systems to interact with a broader environment outside of the AI itself relatively autonomously through a set of tools, such as internet plug-ins. [...] Providing an AI system with a user-interface control would allow it to take actions on sites across the web, not simply retrieve and generate text information. This is signifi cant because soon, frontier AI systems will output not just static language, images, audio, or video, but will likely have the capability to interact with the open internet or user data and applications.⁵
5 Defined using quoted material from: Centre for Security and Emerging Technology., ‘Skating to Where the Puck is Going: Anticipating and Managing Risks from Frontier AI Systems’.
7 Centre for Security and Emerging Technology., ‘Skating to Where the Puck is Going: Anticipating and Managing Risks from Frontier AI Systems’.
Typology of risks³
The work of Laura Weidinger et al. captures the ethical and social risks of harm across a broader spectrum of modalities than are considered in this work.
3 The safety risks of increasingly advanced AI systems are explored in detail in the report from the Centre for Security and Emerging Technology., ‘Skating to Where the Puck is Going: Anticipating and Managing Risks from Frontier AI Systems’.
Discrimination, exclusion and toxicity
Malicious uses
Misinformation harms
Information hazards
Human-computer
interaction harms
Automation, access and environmental harms
AI accountability and oversight infrastructure is nascent
The lack of established testing, accountability and oversight mechanisms was identified as a risk, albeit one that is being addressed. For policymakers, achieving alignment on the right AI accountability structures, including evaluation, access and disclosure processes, is essential.
The appropriate alarm structures for when a dangerous capability is emerging was also discussed. Time is a key variable here. Decision-makers should disambiguate between two separate timelines: 1) when the capability first emerges and 2) when it actually causes significant harm. This distinction is critical when allocating responsibility because policy responses to AI harms will be more dependent on the second timeline (when actual harm is expected to occur) than the first (when the capability has been discovered but has not yet led to harm). Without sufficient oversight and attention to this emergency timeline, policymakers leave societies vulnerable to harm.
“The use of AI undoubtedly poses an array of complex challenges, but policymakers should not be dissuaded from taking action to address emerging concerns by supposed tensions between innovation and safety, the evolving nature of the field, or the relatively nascent mechanisms for accountability.”
Institute for Advanced Studies IAS, Comment of the AI Policy and Governance Working Group on the NTIA AI Accountability Policy Request for Comment
Participants strongly encouraged policymakers to monitor the development of AI systems that may be able to recreate themselves without human oversight (also known as autonomous replication). While model evaluations or ‘dangerous capability evaluations’ were discussed as an important way to interrogate AI systems, a warning emerged from the roundtables not to rely too heavily on their results. Evaluations are usually designed to investigate one particular risk or element, meaning that worrying model competencies outside of that scope might be missed.
The fast tempo of AI progress means that even a shared language for grappling with policy challenges is still emerging. Certain key terms have been ushered into use before they’ve been fully defined. For example, there’s currently no agreed definition for the term ‘frontier models,’ while ‘data equity’ can be understood in a variety of ways. Even apparently self-explanatory terms, like ‘education’ were questioned during the roundtables. For example, what does it mean to be educated and does this change as society changes?